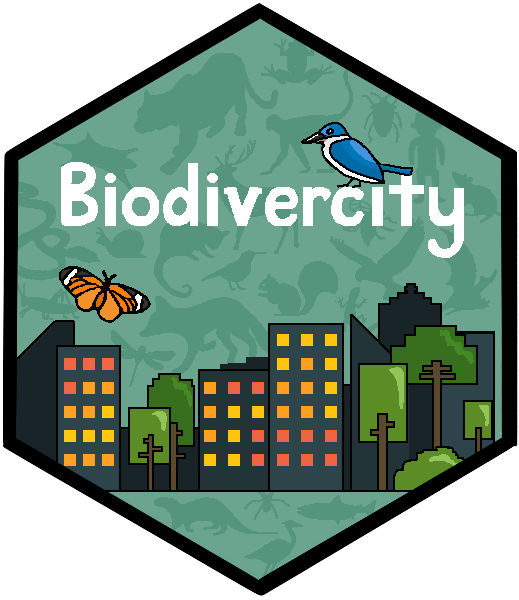
Animals: Simulate Missing Surveys
Visualise the reduction in number of animal species if surveys are missed
2022-12-08
Source:vignettes/more/animals-simulate-missing-surveys.Rmd
animals-simulate-missing-surveys.Rmd
Despite best efforts to ensure consistency in sampling, there may be situations where surveys are missed. For example, animal surveys could not be performed due to movement restrictions when the COVID-19 pandemic hit Singapore.
Simulations can be performed to observe the loss in species richness
(number of species) if surveys are missed. The following article shows
an example if 15
surveys conducted within the Punggol
(PG
) area are randomly excluded from Survey Cycle
5
, during Survey Period 1
(March – April
2017). This corresponds to the same time-of-year in Survey Period
2
(March – April 2020) when 15 bird surveys were missed at
Punggol, due to COVID-19 movement restrictions.
First, load the necessary packages to run the analysis:
library("biodivercity")
library("ggplot2") # for visualisation
Run simulation
Load and process the example datasets, then run the function
exclude_simulator()
.
data("animal_observations")
data("animal_surveys")
# filter animal observations to taxon of interest
birds <- filter_observations(observations = animal_observations,
survey_ref = animal_surveys,
specify_taxon = "Aves", # birds
specify_area = "PG", # punggol
specify_period = "1") # survey period 1
# convert animal observations to community matrix
birds <- as.data.frame.matrix(xtabs(abundance ~ survey_id + species, data = birds))
birds <- cbind(survey_id = rownames(birds), birds) # convert rownames to col
set.seed(123)
scenarios <-
exclude_simulator(birds, animal_surveys,
exclude_num = 15, exclude_level = "survey", specify_cycles = 5)
scenarios
a tabulation of results from each simulated
iteration (column). The count of sampling points with each species (row)
is shown. The column full
shows the breakdown of counts
within the original dataset.
species | full | iter1 | iter2 | iter3 | iter4 | iter5 | iter6 | iter7 | iter8 | iter9 | iter10 | iter11 | iter12 | iter13 | iter14 | iter15 | iter16 | iter17 | iter18 | iter19 | iter20 | iter21 | iter22 | iter23 | iter24 | iter25 | iter26 | iter27 | iter28 | iter29 | iter30 | iter31 | iter32 | iter33 | iter34 | iter35 | iter36 | iter37 | iter38 | iter39 | iter40 | iter41 | iter42 | iter43 | iter44 | iter45 | iter46 | iter47 | iter48 | iter49 | iter50 | iter51 | iter52 | iter53 | iter54 | iter55 | iter56 | iter57 | iter58 | iter59 | iter60 | iter61 | iter62 | iter63 | iter64 | iter65 | iter66 | iter67 | iter68 | iter69 | iter70 | iter71 | iter72 | iter73 | iter74 | iter75 | iter76 | iter77 | iter78 | iter79 | iter80 | iter81 | iter82 | iter83 | iter84 | iter85 | iter86 | iter87 | iter88 | iter89 | iter90 | iter91 | iter92 | iter93 | iter94 | iter95 | iter96 | iter97 | iter98 | iter99 | iter100 |
---|---|---|---|---|---|---|---|---|---|---|---|---|---|---|---|---|---|---|---|---|---|---|---|---|---|---|---|---|---|---|---|---|---|---|---|---|---|---|---|---|---|---|---|---|---|---|---|---|---|---|---|---|---|---|---|---|---|---|---|---|---|---|---|---|---|---|---|---|---|---|---|---|---|---|---|---|---|---|---|---|---|---|---|---|---|---|---|---|---|---|---|---|---|---|---|---|---|---|---|---|---|
Acridotheres javanicus | 36 | 36 | 36 | 36 | 36 | 36 | 36 | 36 | 36 | 36 | 36 | 36 | 36 | 36 | 36 | 36 | 36 | 36 | 36 | 36 | 36 | 36 | 36 | 36 | 36 | 36 | 36 | 36 | 36 | 36 | 36 | 36 | 36 | 36 | 36 | 36 | 36 | 36 | 36 | 36 | 36 | 36 | 36 | 36 | 36 | 36 | 36 | 36 | 36 | 36 | 36 | 36 | 36 | 36 | 36 | 36 | 36 | 36 | 36 | 36 | 36 | 36 | 36 | 36 | 36 | 36 | 36 | 36 | 36 | 36 | 36 | 36 | 36 | 36 | 36 | 36 | 36 | 36 | 36 | 36 | 36 | 36 | 36 | 36 | 36 | 36 | 36 | 36 | 36 | 36 | 36 | 36 | 36 | 36 | 36 | 36 | 36 | 36 | 36 | 36 | 36 |
Acridotheres tristis | 21 | 20 | 21 | 20 | 21 | 20 | 21 | 20 | 21 | 20 | 21 | 21 | 21 | 21 | 21 | 20 | 21 | 20 | 20 | 21 | 21 | 21 | 21 | 21 | 20 | 21 | 21 | 20 | 21 | 20 | 20 | 21 | 21 | 20 | 21 | 21 | 20 | 21 | 21 | 21 | 21 | 20 | 20 | 20 | 20 | 20 | 20 | 20 | 21 | 21 | 21 | 21 | 21 | 20 | 20 | 21 | 21 | 20 | 21 | 20 | 21 | 20 | 20 | 21 | 21 | 21 | 21 | 20 | 20 | 20 | 20 | 20 | 21 | 20 | 21 | 20 | 20 | 20 | 20 | 20 | 20 | 21 | 21 | 20 | 21 | 21 | 21 | 21 | 21 | 20 | 21 | 21 | 20 | 20 | 21 | 20 | 20 | 21 | 21 | 21 | 20 |
Acrocephalus bistrigiceps | 1 | 1 | 1 | 1 | 1 | 1 | 1 | 1 | 1 | 1 | 1 | 1 | 1 | 1 | 1 | 1 | 1 | 1 | 1 | 1 | 1 | 1 | 1 | 1 | 1 | 1 | 1 | 1 | 1 | 1 | 1 | 1 | 1 | 1 | 1 | 1 | 1 | 1 | 1 | 1 | 1 | 1 | 1 | 1 | 1 | 1 | 1 | 1 | 1 | 1 | 1 | 1 | 1 | 1 | 1 | 1 | 1 | 1 | 1 | 1 | 1 | 1 | 1 | 1 | 1 | 1 | 1 | 1 | 1 | 1 | 1 | 1 | 1 | 1 | 1 | 1 | 1 | 1 | 1 | 1 | 1 | 1 | 1 | 1 | 1 | 1 | 1 | 1 | 1 | 1 | 1 | 1 | 1 | 1 | 1 | 1 | 1 | 1 | 1 | 1 | 1 |
Actitis hypoleucos | 1 | 1 | 1 | 1 | 1 | 1 | 1 | 1 | 1 | 1 | 1 | 1 | 1 | 1 | 1 | 1 | 1 | 1 | 1 | 1 | 1 | 1 | 1 | 1 | 1 | 1 | 1 | 1 | 1 | 1 | 1 | 1 | 1 | 1 | 1 | 1 | 1 | 1 | 1 | 1 | 1 | 1 | 1 | 1 | 1 | 1 | 1 | 1 | 1 | 1 | 1 | 1 | 1 | 1 | 1 | 1 | 1 | 1 | 1 | 1 | 1 | 1 | 1 | 1 | 1 | 1 | 1 | 1 | 1 | 1 | 1 | 1 | 1 | 1 | 1 | 1 | 1 | 1 | 1 | 1 | 1 | 1 | 1 | 1 | 1 | 1 | 1 | 1 | 1 | 1 | 1 | 1 | 1 | 1 | 1 | 1 | 1 | 1 | 1 | 1 | 1 |
Aegithina tiphia | 13 | 12 | 13 | 12 | 13 | 12 | 13 | 12 | 13 | 12 | 13 | 13 | 13 | 13 | 13 | 12 | 13 | 12 | 12 | 13 | 13 | 13 | 13 | 13 | 12 | 13 | 13 | 12 | 13 | 12 | 12 | 13 | 13 | 12 | 13 | 13 | 12 | 13 | 13 | 13 | 13 | 12 | 12 | 12 | 12 | 12 | 12 | 12 | 13 | 13 | 13 | 13 | 13 | 12 | 12 | 13 | 13 | 12 | 13 | 12 | 13 | 12 | 12 | 13 | 13 | 13 | 13 | 12 | 12 | 12 | 12 | 12 | 13 | 12 | 13 | 12 | 12 | 12 | 12 | 12 | 12 | 13 | 13 | 12 | 13 | 13 | 13 | 13 | 13 | 12 | 13 | 13 | 12 | 12 | 13 | 12 | 12 | 13 | 13 | 13 | 12 |
Aerodramus germani | 1 | 1 | 1 | 1 | 1 | 1 | 1 | 1 | 1 | 1 | 1 | 1 | 1 | 1 | 1 | 1 | 1 | 1 | 1 | 1 | 1 | 1 | 1 | 1 | 1 | 1 | 1 | 1 | 1 | 1 | 1 | 1 | 1 | 1 | 1 | 1 | 1 | 1 | 1 | 1 | 1 | 1 | 1 | 1 | 1 | 1 | 1 | 1 | 1 | 1 | 1 | 1 | 1 | 1 | 1 | 1 | 1 | 1 | 1 | 1 | 1 | 1 | 1 | 1 | 1 | 1 | 1 | 1 | 1 | 1 | 1 | 1 | 1 | 1 | 1 | 1 | 1 | 1 | 1 | 1 | 1 | 1 | 1 | 1 | 1 | 1 | 1 | 1 | 1 | 1 | 1 | 1 | 1 | 1 | 1 | 1 | 1 | 1 | 1 | 1 | 1 |
Visualise results
These 100 bootstrapped scenarios may be used in further analyses, or visualised to observe the variation in species richness within the sampled area of interest (Punggol):
spp_richness <- colSums(!is.na(scenarios[,-1])) # calculate total no. of bird species per scenario
spp_richness <- spp_richness %>%
as.data.frame() %>%
dplyr::rename(n = ".") %>%
dplyr::mutate(full = nrow(scenarios)) %>%
dplyr::mutate(area = "Punggol")
spp_richness %>%
ggplot(aes(x = area, y = n)) +
geom_boxplot() +
geom_point(aes(y = full), shape=4, color = "blue") +
stat_summary(fun = mean, geom = "point", shape = 5) +
xlab("") + ylab("No. of species") +
theme_bw()
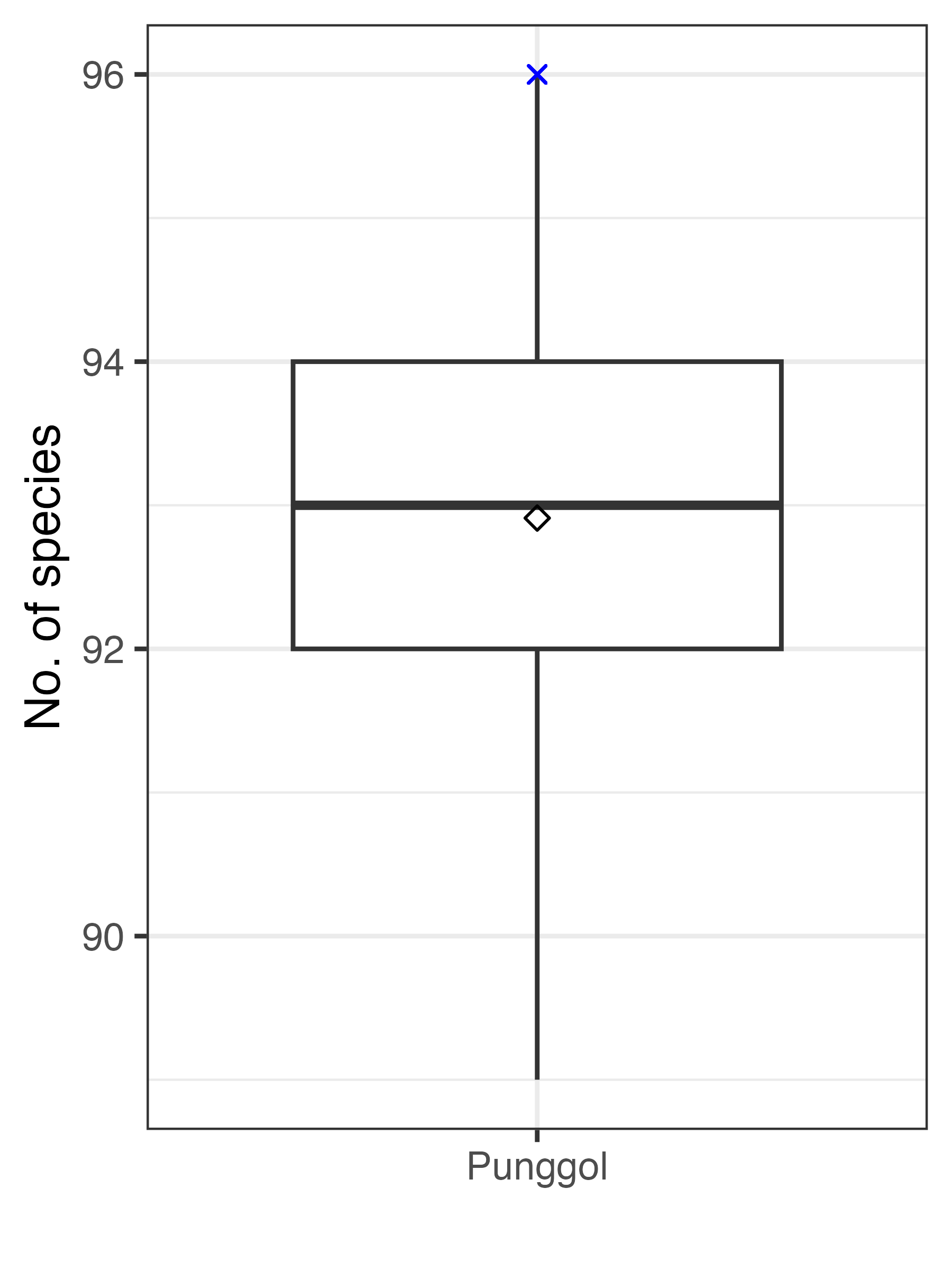
Figure: Number of bird species at Punggol, Singapore if 15 surveys were missed in Survey Cycle 5 (March – April 2017). The blue cross denotes the count in full dataset, while the diamond symbol denotes the mean count across 100 scenarios where survey data were randomly excluded.
Visualisations can also be performed at the level of individual species. Species that are relatively less common are more likely to be missed at sampling points:
# transform for plotting
plot_bird_spp <- scenarios %>%
tidyr::pivot_longer(cols = num_range("iter", 1:ncol(scenarios)),
names_to = "iteration", values_to = "n") %>%
dplyr::mutate(taxon = "Aves")
# get order of species based on total count
species_order <- plot_bird_spp %>%
dplyr::group_by(species) %>%
dplyr::summarise(order = mean(full))
plot_bird_spp %>%
dplyr::left_join(species_order) %>%
ggplot(aes(x = reorder(species, order), y = n)) +
geom_boxplot() +
geom_point(aes(y = full), shape = 4, color = "blue") +
stat_summary(fun = mean, geom = "point", shape = 5) +
xlab("") + ylab("No. of sampling points") +
theme_bw() +
coord_flip()
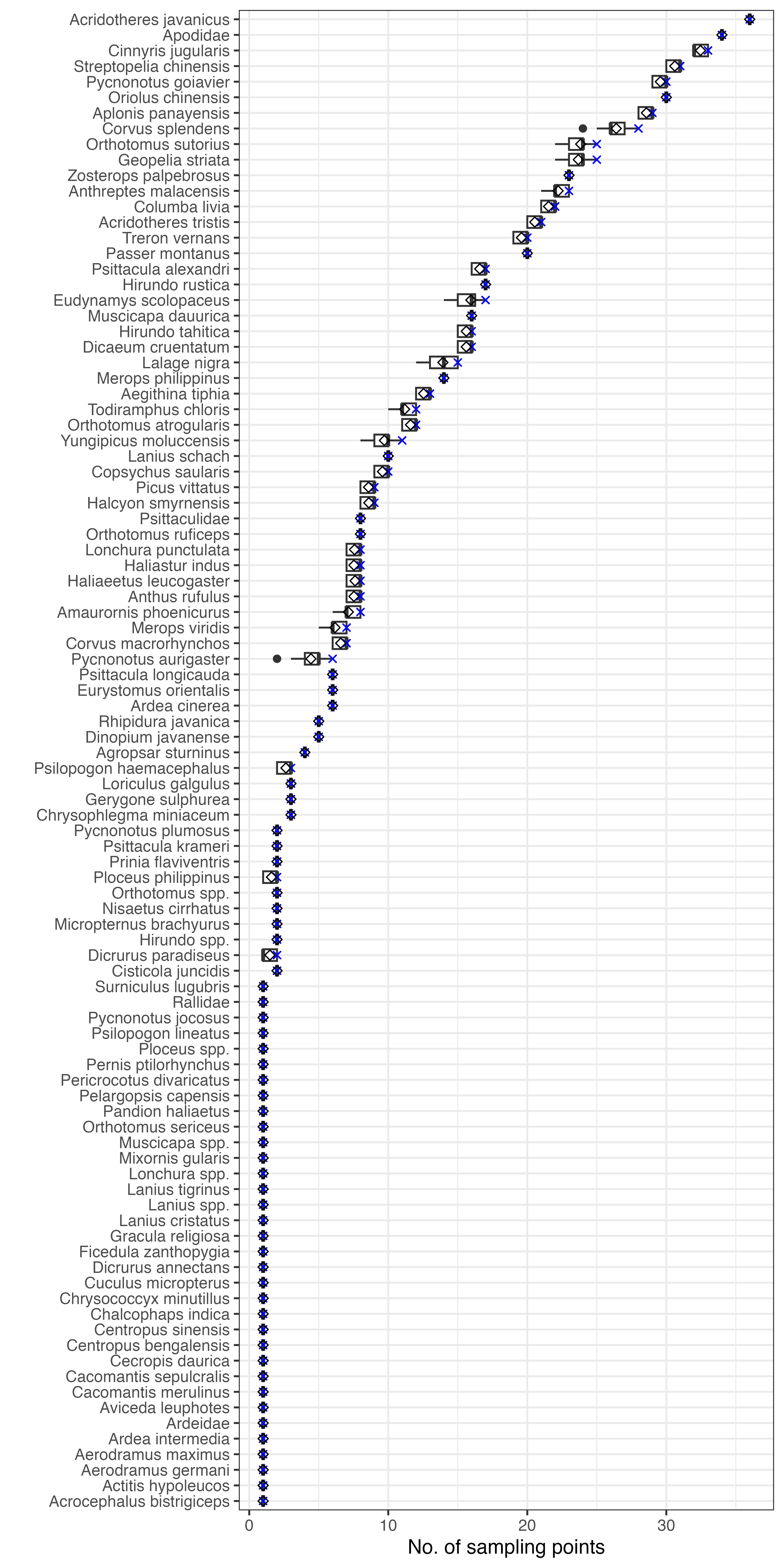
Figure: Number of sampling points that bird species were observed at Punggol, Singapore if 15 surveys were missed in Survey Cycle 5 (March – April 2017). The blue cross denotes the count in full dataset, while the diamond symbol denotes the mean count across 100 scenarios where survey data were randomly excluded. Species are arranged in descending order of counts.